How Cushman & Wakefield Services Is Leading Its Customers Into the Future of Reliability
To reduce unplanned downtime for a leading Fortune 500 pharmaceutical client, Cushman & Wakefield (C&W) Services moved from a traditional route based condition monitoring program to a wireless and artificial intelligence (AI) based predictive maintenance (PdM) program. The shift in technology not only led to reduced unplanned downtime, but also a better utilization of resources and a deeper understanding of asset condition. Here’s how C&W Services approached the challenges and arrived at solutions.
Background
C&W Services is an integrated facilities services and management company that serves more than 600 clients through the maintenance of more than 600 million square feet across North America. It transforms clients’ maintenance practices from reactive to predictive. One of its clients, a large pharmaceutical Fortune 500 company, had over 350 critical assets, including air handling units (AHU), chillers, exhaust fans, and compressors, spread across 40 buildings. C&W Services was using a manual walk-around program, where a handheld data logger collected data every 30 days and sent it to a third-party for analysis.
Challenges
While the walk-around program seemed promising initially, it had several challenges that made it difficult to achieve the reliability goals of the site. Among them:
1. Unplanned Downtime: Infrequent data collection led to failures happening between the monthly walk-around cycles. Even with a well-trained workforce, failures developed quickly and were difficult to catch in time, leading to unplanned downtime.
2. Limitations of Resources: Like many facilities, the pharmaceutical company had too many assets for manual data collection to be effective, requiring more people than were available. Readings were often taken by different people, which led to inconsistencies in data collection.
3. Safety and Accessibility Concerns: Some areas housing critical machinery posed dangers to the technicians collecting data. There were several assets, such as the AHUs, that were not readily accessible because they were behind metal structures and cages.
4. Variable Operating Conditions: The operating parameters of the AHUs were often modified to adapt to external weather conditions. These variable operating conditions led to inaccurate conclusions and an incomplete picture. For instance, different operating conditions made it impossible to trend over time.
All of these factors led to a catastrophic failure of a 200 hp AHU at the research facility. In addition to downtime, the pharmaceutical company incurred $30,000 in repair costs alone.
Goals
The catastrophic failure of the AHU and limitations posed by the walk-around program prompted C&W Services to evaluate wireless PdM solutions available on the market. Its team of reliability engineers set forth the following criteria:
1. Continuous Monitoring of Critical Assets: This allowed for more frequent data collection to avoid machine failures arising between data collection cycles. The team was looking for technology that would automate this and eliminate challenges around variable operating conditions, consistency of readings and manpower shortages.
2. Access to Real-Time Data Insights: With the advent of Industrial Internet of Things (IIoT) and machine learning capabilities, the team was looking for a system to help with anomaly detection capabilities and access to real-time data insights and diagnostics.
3. Safety and Productivity of Personnel: To eliminate hazards associated with a walk-around process and to reallocate manual labor to more productive activities, the team wanted to use wireless sensors for data collection.
4. Ease of Installation: It was necessary for the PdM system to be easy to deploy and quick to implement without the expense of wiring traditional sensors to equipment or undertaking any custom information technology (IT) projects.
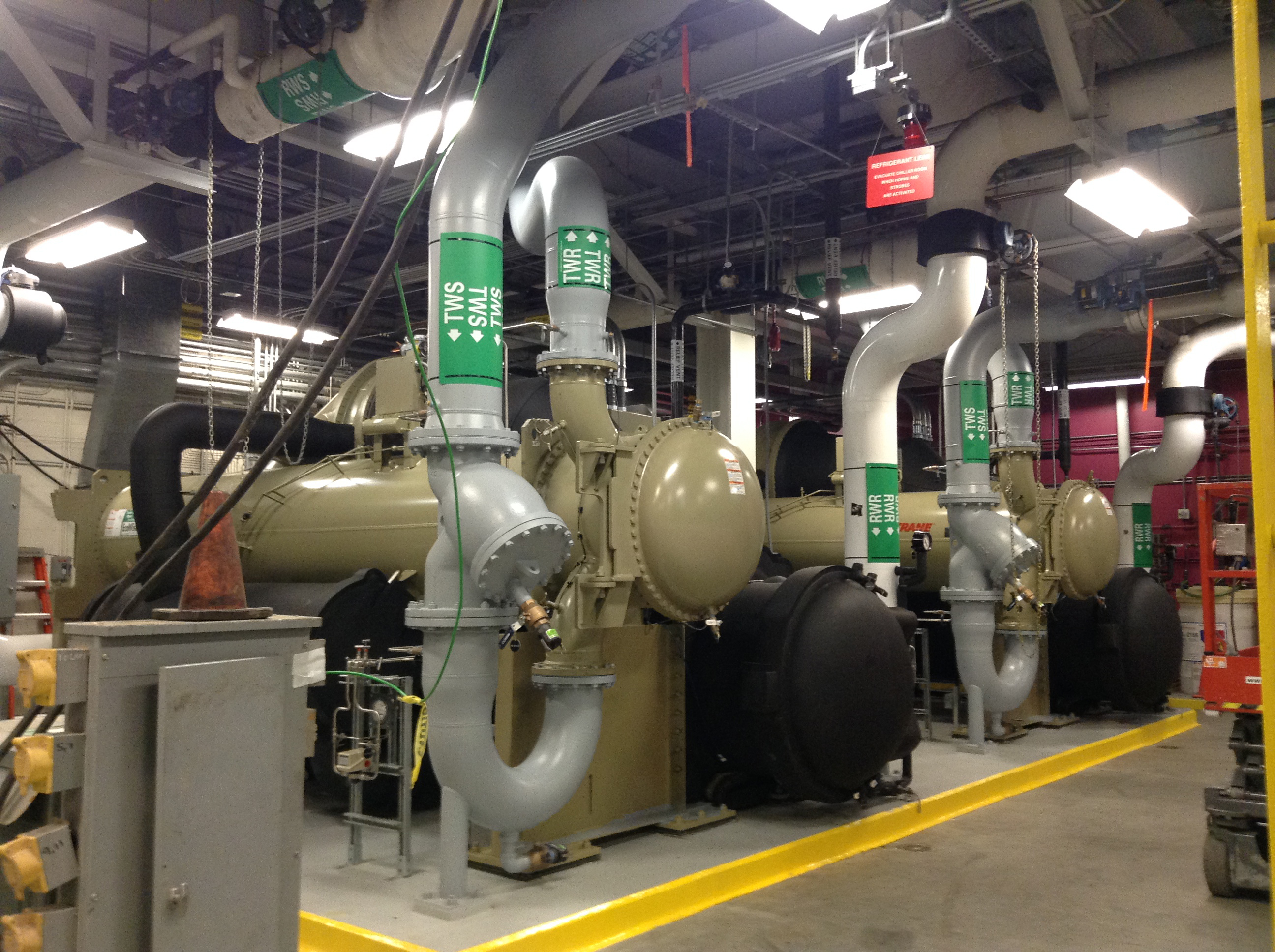
The Solution
After a thorough evaluation, C&W Services selected Petasense, an IIoT predictive maintenance start-up to provide an Asset Reliability and Optimization (ARO) system to help monitor, analyze and predict the health of critical industrial equipment. The system includes wireless sensors, machine learning based analytics and Web and mobile dashboards.
Vibration Motes
Wireless, battery-powered triaxial vibration sensors called motes were mounted on all critical rotating machinery, including AHUs, pumps, compressors, exhaust fans and chillers, to continuously monitor their health. These motes detect defects, like machine imbalance, shaft misalignment, structural looseness, pump cavitation and bearing wear. They connect wirelessly to the Cloud for advanced predictive analytics.
Predictive Analytics
The implementation included predictive analytics that use machine learning and multi-parametric analysis to make predictions about machine failure. By incorporating multiple parameters, such as temperature, pressure, current and ultrasound, a more robust prediction can be made. The IIoT predictive maintenance system provided a machine health score for each machine based on deviations from a multidimensional baseline, providing real-time notifications when the score fell below an acceptable number. This enabled C&W Services to quickly identify problem equipment.
Web and Mobile Dashboards
The ARO system is accessible anytime, anywhere, through a Web or mobile app dashboard. It provides a role-based interface that is customized according to the role. For example, managers are shown only the most essential information, while engineers and analysts get highly interactive charts and rich analysis tools.
Benefits
The benefits were evident soon after installation. C&W Services was able to catch a defect in an AHU that showed high vibration harmonics. An investigation determined looseness in the belts and shaft misalignment. The AHU was shut down and repaired without any unplanned downtime at a much lower cost than if it had failed.
On another AHU, the weekly vibration level was trending up, but when looking at real-time data in parallel with operational data, it was evident the cycling was due to normal workweek variations. C&W Services would not have been able to discover this with periodic readings, nor would it be able to derive a deeper understanding of asset operation cycles and the impact on maintenance.
By implementing wireless PdM on the pharmaceutical company’s critical equipment, C&W Services was able to achieve the goals of increased reliability, reduced failures and ensuring personnel safety. Its team was better equipped to catch defects with real-time, actionable intelligence. This resulted in a substantial cost reduction associated with unnecessary reactive maintenance.
Another huge value driver was the ease of installation and implementation. According to David Auton, senior director of reliability engineering at C&W Services, “Any facility operator can easily deploy PdM, use it effectively, conduct waveform and data analysis, and derive meaningful insights without any formal training.”
Conclusion
The AI-based predictive maintenance implementation helped C&W Services streamline data collection, improve uptime and reduce maintenance costs for a very important pharmaceutical client.
Predictive maintenance is currently one of the biggest uses for IIoT. With advances in wireless connectivity, cloud computing and artificial intelligence, along with lower sensor costs, there is a shift toward wireless condition monitoring. Facility managers have used traditional condition monitoring for decades, but are starting to recognize the benefits of applying wireless technology to bring more value to their clients.