The idea that smart factory technology will displace humans has generated considerable discussion. In a July 2016 report, McKinsey & Company estimates that “
59 percent of all manufacturing activities could be automated.”1 In an article that can be applied to the field of industrial analytics, the MIT Technology Review2 suggests that unlike past experience, technologies are providing solutions that are more humanlike and could, therefore, eliminate jobs that so far have withstood automation.
Given the uncertain outlook, let’s review innovations in machine learning for predictive analytics and analyze the potential impact of maintenance activities.
Background: Machine Learning for Predictive Analytics
The application of machine learning to industrial analytics is part of the technological transformation termed Industry 4.0 or the Industrial Internet of Things (IIoT). Theoretical concepts that were previously limited to the narrow confines of academia are being commercialized rapidly.
Machine learning uses artificial intelligence to detect abnormal industrial machine degradation and failure before they occur. Vast amounts of data generated from sensors embedded in plant equipment are analyzed in real time. Algorithms are trained to identify anomalous sensor behavior or patterns of anomalous behavior. Based on these analyses, time to failure (TTF) and root cause failure analysis (RCFA) are provided.
Within the marketplace, there are numerous commercial solutions based on different approaches to machine learning. The most well-known is supervised machine learning. With supervised machine learning, the algorithm is “trained” on each failure pattern. When a new failure pattern is analyzed, the algorithm labels the pattern based on its prior training.
Unsupervised machine learning is an alternative whereby the learning algorithm does not need to be trained with data labels. Instead, vast amounts of data are analyzed and the algorithm itself generates the label. It is considered a more robust methodology because few resources are required for training the algorithm.
Regardless of methodology, machine learning for industrial analytics is a transformative technology that upends existing maintenance processes.
Industrial Analytics versus Reactive Maintenance
Although industrial plants are not always forthcoming with this information, it is estimated that at least half of all maintenance activities occur only after asset failure is imminent or has already occurred. Reactive maintenance is the most expensive form of repair because there is insufficient time to schedule repairs. Delays can be caused by several factors, including:
- Traveling to the repair site;
- Delays for spare parts or rental equipment;
- Repair instructions or receiving relevant documentation;
- Waiting for machinery to be shut down before work can commence;
- Additional delays for support to arrive at job site.
Reactive maintenance occurs under the pressure of a downtime incident. A delay in returning machinery to its useful state can result in lost productivity and revenue. In some cases, the pressure leads to mistakes or quick fixes. Without the benefit of RCFA, crews often rely on clumsy trial and error approaches.
With machine learning for predictive maintenance, early warning and time to failure are provided for degradation or failure. Production loads can be slowed while spare parts are ordered and scheduling is optimized. With the benefit of RCFA, most guesswork can be eliminated.
The result? Less disruptive maintenance and limited asset downtime.
Industrial Analytics versus Preventive Maintenance
Regularly scheduled preventive maintenance (PM) is based on time or equipment usage. PM scheduling is determined by:
- Original equipment manufacturer (OEM) guidelines as per equipment manuals;
- Mean time between failures (MTBF) probability estimates;
- Regulatory compliance;
- Equipment condition;
- Schedule optimization.
With regularly scheduled maintenance, equipment utilization is maintained. However, maintenance activities carry an inherent risk of human error. These include errors in equipment reinstallation or reassembly, mistakes that damage the asset undergoing repair or adjustment and failure to follow best practices for repairs.
According to a study
3 on fossil fuel power plants, the majority of maintenance outages occurred in less than a week after a maintenance outage (1,772 of 3,146 maintenance outages occurred after a planned or forced maintenance outage). The conclusion was that “in 56 percent of the cases, unplanned maintenance outages were caused by errors committed during a recent maintenance outage.”
One of the underlying assumptions for preventive maintenance is that as assets age, they wear out. However, research suggest that only 11 percent of maintenance repairs are based on age-related factors. Most asset failures are considered random and are not associated with a predefined failure pattern.
Finally, there are costs associated with planned machine shutdown. These include the direct cost (e.g., labor, spare part, etc.) and the economic cost from lost production.
Machine learning for predictive asset maintenance, on the other hand, can eliminate or reduce unnecessary preventive maintenance. This is because with machine learning, maintenance is based on an algorithm that triggers an alert of an evolving failure event. With RCFA, maintenance is targeted to the specific equipment that requires repair.
Preventive maintenance applies man-made rules for maintenance that are based on historic data, factory maintenance practices, or even scheduling convenience. It is not a precise discipline and, as a result, it is the cause of significant instances of over maintenance, under maintenance, or faulty maintenance.
Figure 1: Well-known solutions based on supervised machine learning (Source: Presenso)

Figure 2: Reactive maintenance occurs under the pressure of a downtime incident (Source: Presenso)
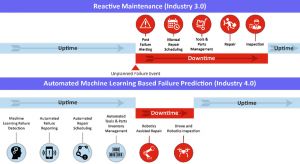
Figure 3: Age related versus no age related failure patterns

How Machine Learning for Predictive Maintenance Impacts Employment
In demonstrating the advantages of machine learning for predictive maintenance, the assumption is that over time, there will be a shift toward this solution type and a reduction in both reactive and unnecessary preventive maintenance.
Expectations point to a drop in the overall level of employment in operations and maintenance (O&M), with no significant areas of potential offsetting increases.
The
Journal of Business and Media Psychology suggests that, “maintenance would likely be subject to further automation, whereby increasing complexity arising from integrated process and system architectures would most probably require more demand for cross-functional management control capabilities and capacities in troubleshooting and improvisation.”4 It is likely that in the era of Industry 4.0, maintenance workers will require greater levels of skills and training, especially when robotics and automation become standardized within the maintenance mix. Nevertheless, at the current pace of innovation and investment, the overall number of O&M workers is likely to decline.
New Roles in the Smart Factory
If workers are replaced, will new roles emerge?
The
Boston Consulting Group (BCG) suggests that new jobs will emerge, such as robotics coordinator and industrial data scientist. At the same time, existing jobs, such as assembly line worker, service technician and machine operator, will change. BCG’s expectation is that a machine operator “will require less machine and product specific training, but will need enhanced capabilities for utilizing digital devices and software and accessing a digital knowledge repository.”5
Will Data Scientists Replace Factory Workers?
Many analysts expect a significant demand for industrial data scientists. The BCG study assumes there will be a need for more data scientists and lumps together several big data processes for this role: extracting data, analyzing data, identifying correlations and drawing conclusions on RCFA.
However, another school of thought believes this commonly held view is based on outdated assumptions about the role of Industry 4.0 technology. Simply stated, technology solutions based on big data will replace the need for facility level or even remote industrial data scientists. The quantity and scope of big data that forms the basis of an industrial analytics solution exceeds the bandwidth of internal resources and requires a dedicated technology platform. Solutions that are applicable to industrial plants need to be easily accessible and visualize asset degradation without the input of plant experts.
According to
one study6, 40 percent of companies are struggling to recruit and retain data analytics employees. With no obvious solution to this labor shortage, many industrial plants will even lack the option of competing for this limited pool of talent. Ultimately, market forces will shift the burden from internal resources to third-party technological solutions that require limited in-house expertise.
Overemployment or Underemployment? Perhaps Both
A
research paper published by professors from the University of Bath and the University of Liverpool reaches a disturbing conclusion:
“At present, educational systems appear not to be adapting fast enough to respond to future labor demands imposed by Industry 4.0. If not addressed, this challenge may result in the required skills being undersupplied, thereby fueling disparities between labor supply and demand, which consequently may cause unemployment levels to rise.”
7
The rise of automation will eliminate repetitive and manual tasks, ranging from production line to maintenance. The World Economic Forum released a comparison of the Top 10 job skills needed in 2015 versus 2020, as shown in Table 1.
Table 1 – Top 10 Skills
2020
|
2015
|
---|
1
|
Complex Problem-Solving
|
1
|
Complex Problem-Solving
|
2
|
Critical Thinking
|
2
|
Coordinating with Others
|
3
|
Creativity
|
3
|
People Management
|
4
|
People Management
|
4
|
Critical Thinking
|
5
|
Coordinating with Others
|
5
|
Negotiation
|
6
|
Emotional Intelligence
|
6
|
Quality Control
|
7
|
Judgment / Decision-Making
|
7
|
Service Orientation
|
8
|
Service Orientation
|
8
|
Judgment / Decision-Making
|
9
|
Negotiation
|
9
|
Active Listening
|
10
|
Cognitive Flexibility
|
10
|
Creativity
|
(Source: The Future of Jobs Report, World Economic Forum)
Even during this short time period, there is an increased expectation for skills, such as creativity, emotional intelligence and cognitive flexibility, and a drop in need for quality control, negotiation and coordinating with others.
Although this study is directional and not specific to Industry 4.0, it points to the growing need for soft skills, such as critical thinking. The future maintenance worker will not be a data scientist, but he or she will need to interpret machine-generated big data analysis.
Realistically, manufacturers cannot rely on educational institutions to fill the skill set gap and will need to find ways to reskill their employees and equip them with the tools needed to succeed. In this way, the efficiencies gained by IIoT predictive maintenance can offset the job roles required for the smart factory.
Conclusion
In the new era of Industry 4.0, a shift from reactive to predictive maintenance is now technologically feasible. Furthermore, many preventive maintenance practices need updating. Change will not happen overnight and as long as there are industrial plants, machinery will break down and humans will be involved in the repair process. However, given the disruptive nature of Industry 4.0 and machine learning, it is likely that maintenance-related employment levels will decline.
References
- https://www.mckinsey.com/business-functions/digital-mckinsey/our-insights/where-machines-could-replace-humans-and-where-they-cant-yet
- https://www.technologyreview.com/s/607850/is-automation-warping-the-labor-market-as-dramatically-as-we-think/
-
https://maintenanceresources.com/referencelibrary/ezine/preventmaint.html
-
http://journal-bmp.de/2015/12/auswirkungen-von-industrie-4-0-auf-menschliche-arbeit-und-arbeitsorganisation/?lang=en
-
https://www.bcgperspectives.com/content/articles/technology-business-transformation-engineered-products-infrastructure-man-machine-industry-4/?chapter=4
-
https://www.cio.com/article/3025869/analytics/6-analytics-trends-that-will-shape-business-in-2016.html
-
https://livrepository.liverpool.ac.uk/3009339/1/C:%5cUsers%5cschaefdk%5cDropbox%5c_SCHAEFER_UNIVERSITY%5c%5b02%5d%20Research%5c%5b03%5d%20Publications%5c%5b02%5d%20Published%5c%5b2017%5d%20%5b139%5d%20%5bCA%5d%20ICMR%20Dirk%20Joe%20I4%20Demographics%5cICMR17_paper_76_final.pdf